Creating a Lookup Table with 3rd Party Data for Automated Enrichment
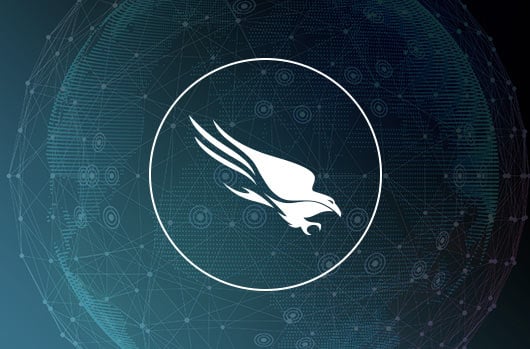
An Autonomous System Number (ASN) is a group of one or more IP prefixes run by one or more network operations that maintain a single, clearly-defined routing policy. DROP is an acronym for Do Not Route or Peer. The Spamhaus ASN-DROP list contains the ASNs that are associated with the worst of the worst behavior. In other words, these are the adversaries that might try to attack you. In this tutorial, you’ll see how to leverage Falcon Next-Gen SIEM with the Spamhaus ASN-DROP list.
Using Spamhaus’ ASN-DROP list, you can block rogue ASNs at the perimeter and hunt for DROP’d ASNs. Falcon Next-Gen SIEM allows you to upload the list as a lookup file, show events that reference them in a table, or view them on a world map.
This quick-start guide demonstrates one simple but powerful use case for Falcon Next-Gen SIEM.
Prerequisites:
- Falcon Insight XDR (Falcon Next-Gen SIEM 10GB Free)
- Python 3
- VS Code (optional)
You’ll learn how an uploaded CSV file can be leveraged as a lookup table in CrowdStrike’s Falcon Next-Gen SIEM. In this example, a Spamhaus ASN-DROP list will be used to create a CSV from their API, which will then be uploaded to Falcon Next-Gen SIEM. Then, you’ll see how to search for IPs that match this lookup table, and visualize them on a world map.
Already have a CSV you want to use as a lookup table? This example will show you how to query against it.
Create a CSV File from the Spamhaus ASN-DROP List
The fastest way to generate a CSV file is with your terminal and vi (or Emacs). If you’d rather not use the terminal, please skip to the Create a CSV File using Jupyter Notebook section.
Most operating systems have Python installed. If yours doesn’t, you can use Homebrew and run brew install python
on macOS. For others, see Python for Beginners.
Open your favorite terminal and create a directory for your code.
mkdir -p dev/next-gen-siem-quickstart cd dev/next-gen-siem-quickstart
Then, set up a Python virtual environment.
python -m venv .venv source .venv/bin/activate
Install the requirements you’ll need to fetch and manipulate the data.
pip install requests pandas
Create an asn_drop.py
file and add the following code to it:
import pandas as pd import requests df = pd.read_json("https://www.spamhaus.org/drop/asndrop.json", lines=True) # drop the last row that includes metadata and the columns inferred for that row df = df.iloc[:-1] df = df.drop(columns=['type', 'timestamp', 'size', 'records', 'copyright', 'terms']) df["asn"] = df["asn"].astype(int) df.to_csv('asndrop.csv', index=False)
Save the file and run the following command to execute its code:
python asn_drop.py
When the command executes successfully, you’ll see a asndrop.csv
file in the next-gen-siem-quickstart
directory. This process will take several seconds to complete.
NOTE: If you see ModuleNotFoundError: No module named 'requests'
, activate your virtual environment:
source .venv/bin/activate
Create a CSV File using Jupyter Notebook
You can also use Jupyter Notebook and VS Code to create your CSV file. After installing VS Code, install the Jupyter extension. You can do this by going to View > Extensions and searching for “jupyter”. Click the Install button to continue.
Next, set up your Python environment:
- Open or create your project directory
- Create an
ASNs.ipynb
file - Go to View > Terminal
- Create and activate a virtual environment:
python -m venv .venv source .venv/bin/activate
Then, install the libraries you’ll need to fetch and process the JSON response.
pip install requests pandas
Add this code to your notebook’s first cell:
import pandas as pd import requests
Select your virtual environment in the top right corner.
Run the code in the first cell (or use Shift + Enter). Add this code to the second cell:
df = pd.read_json("https://www.spamhaus.org/drop/asndrop.json", lines=True) # drop the last row that includes metadata and the columns inferred for that row df = df.iloc[:-1] df = df.drop(columns=['type', 'timestamp', 'size', 'records', 'copyright', 'terms']) df["asn"] = df["asn"].astype(int) df.to_csv('asndrop.csv', index=False)
After several seconds, this code creates an asndrop.csv
file in the current directory. You can see what this notebook looks like in the screenshot below.
Import the Lookup File into Falcon Next-Gen SIEM
Log in to the Falcon platform and go to Next-Gen SIEM > Lookup files. Import the CSV file you created by clicking the Create file button and selecting Import file. Choose the file you created and click Import.
Switch back to the Search tab to continue.
Search Events and Match Against ASN-DROP List
Copy this CrowdStrike Query Language (CQL) query into the search box.
#type=falcon-raw-data | #event_simpleName = NetworkConnectIP4 | asn(field=RemoteAddressIP4, as=ASN) | match(file="asndrop.csv", field=[ASN.asn], column=asn) | table([ComputerName, aid, RemoteAddressIP4, ASN.asn, domain, cc])
In this query, the type
indicates you’re using the Falcon platform data, the event_simpleName
is looking at IP addresses, and there’s an asn()
function. This function allows you to pull out the ASN information of every external IP address that has been accessed by a Falcon sensor.
The next line starts with match()
and does a comparison of the ASNs in your events with the lookup file that has the worst-behaving ASNs. The last line creates a table with the events that have malicious data that’s connected to those bad ASNs.
In this data, you should look for known bad ASNs that are clearly not your B2B partners.
Another handy tool you can use is the world map visualization. Select this tool from the second dropdown above the search box.
The reason it says Incompatible is because the data is not formatted in a way that it can render the data on a map. To fix this, remove the last line and replace it with the worldMap()
function.
#type=falcon-raw-data | #event_simpleName = NetworkConnectIP4 | asn(field=RemoteAddressIP4, as=ASN) | match(file="asndrop.csv", field=[ASN.asn], column=asn) | worldMap(ip=RemoteAddressIP4)
TIP: If you want to comment out a line so it’s not read, prefix it with //
.
After making this change, run the query, and you should see the events on a map of the world.
What else can you do with this query? That’s a good question! You can save it as a scheduled search, add it to a dashboard, or export the results as a file. Pretty amazing, don’t you think?
Querying Other Data with Falcon Next-Gen SIEM
This is just one example of how you can query data with Falcon Next-Gen SIEM. In this example, we used falcon-raw-data
as the repository. You could also use the okta
repository to find when a user is created.
#repo="okta" #Vendor="okta" #event.module="sso" | #event.kind="event" | event.action="user.lifecycle.create" | table([user.target.full_name,user.target.name,message,user.name,user.target.id,@timestamp], limit=1000)
This allows you to create an Okta event tracking dashboard.
For more query examples, see the Helpful CQL Queries in the LogScale Community Content GitHub repo.
Learn More About Falcon Next-Gen SIEM
This tutorial introduced you to CrowdStrike’s Falcon Next-Gen SIEM and showed you how to:
- Use the
asn()
function with CQL - Find external traffic going to known bad ASNs
- Create and use lookup tables
- Visualize results on a world map
Want to learn more about Falcon Next-Gen SIEM? Visit our Tech Hub’s Next-Gen SIEM category for blog posts, video demos, and hands-on labs.
You might also enjoy the r/crowdstrike community on Reddit, where Cool Query Fridays often feature Falcon Next-Gen SIEM query examples.
Have questions? Connect with us in our Community Forums.