- Noisy alerts caused by an increased number of rules for detecting malicious behavior overburden security analysts.
- Alert fatigue happens when an overwhelming number of alerts desensitize the security team tasked with responding to them.
- CrowdStrike CrowdScore™ automatically prioritizes activity that is more unusual in part by noticing which alerts are noisy in each environment.
Current Practices in Dealing With Alert Fatigue
Let us start by exploring alert fatigue and how the industry has been responding to this challenge. Alert fatigue is when an overwhelming number of alerts desensitizes the team tasked with responding to them; this commonly leads to missing, ignored, or delayed responses. Over the years, the security industry has developed several standard practices to respond to the alert fatigue challenge. The most common are detection filtering, capacity management and prioritized detection. Taking a closer look at these practices allows us to understand how they may impact security operation detection capabilities. Detection filtering is the concept of applying filtering (i.e., allowlist or exclusion rules) to your detection corpus. Through this process, logic is effectively muted or removed from the detection corpus, leading to a decrease in visibility. We'll explore this concept later in the article when we discuss the concept of a detection funnel.
Capacity management is the process of assessing the number of detection alerts produced over a period of time and how fast these alerts can be investigated and resolved. As the security maturity of the organization goes up, so does the size of your detection corpus. To keep up with an increased number of alerts and hence continue to detect and respond promptly, the size of the security operation team has to expand. This can lead to challenges such as attracting, developing, and retaining talent and ultimately to a linear operational cost increase. Prioritized detection considers some of the shortcomings of the capacity management approach and recognizes that team size cannot scale at the same speed as your detection corpus. To continue to focus on what is expected to be good detection logic, a priority system is applied to detections. It is not uncommon in the security operations world to have detections tightly connected with response activities. This process leads to a response queueing approach, where detections with a lower priority are ignored or queued and delayed until a sufficient workforce can handle the load. The above approaches have been adopted by many. While they can appear as an effective way to deal with the alert fatigue challenge, they also increase your operational risks and cost.
What if there was a better way to manage and develop your detection corpus while avoiding alert fatigue and its consequences?
The Detection Funnel Paradox
A brief web search about how security operations build detection capabilities will quickly surface the ubiquitous "funnel" approach to detection, closely related to the so-called “Pyramid of Pain.”But this simple approach, sometimes couched as cutting edge or a best practice, is just a natural outcome of evolutionary processes that have existed at least since Snort rules dominated the prehistoric security operations landscape. The more general the detect, the noisier it becomes, and the smaller the fraction of those false positive prone detects that uncover real attacks. The more false positives, the more pressure from analysts to disable the detect.
The final result is that the noisiest detections get turned off. "The funnel" is just another way of saying we asked detection engineers to write many rules that are now wasted effort because no analysts are looking at them. Creating exceptions or allowlisting is another form of tuning that we will examine here. There's not much new to say about managing unwieldy repositories of detection logic that hasn't already been the subject of 30 years of hand-wringing. The underlying issues need to be identified, and a different approach is required to get us off the detect treadmill.
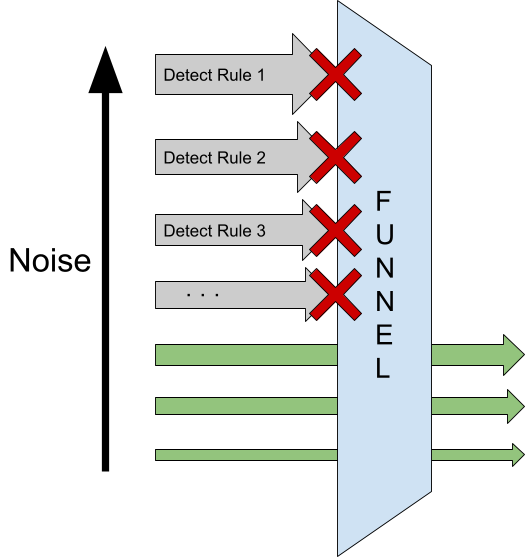
a way to determine when to pay attention to noisier rules, and surface them as alerts. And as we dig deeper, we find that the problem gets much worse. Less frequent alerts tend to be brittle — they tend to detect a particular, narrow set of attack techniques that fall out of favor with attackers over time. They are reactive, detecting only specific aspects of known recent attacks with little chance of detecting tomorrow's attacks. As a result, those brittle detects need to be replaced by new rules that detect new attacker behaviors. On the other hand, identifying more general behaviors tends to accrue new false positives, requiring constant effort to maintain or "tune" them according to the funnel model. This effort represents another cost in person-hours. The result is a solution that scales poorly and eventually places a ceiling on how many detect rules we can afford to show our analysts and how many we can afford to maintain. As the environment changes, the rules need tuning. As our detection corpus grows, the overall tuning load increases proportionally, or some rules must be culled from the detection corpus. As attacker behavior changes, new rules need to be developed, which increases the detection corpus maintenance workload. The growth of enterprise environments results in a proportional increase in the number of alerts that have to be triaged. The fact that there are so many different axes for noise to increase over inevitably leads to the collapse of the funnel model. The funnel simply does not scale. Clearly, we need an alternative to the funnel model. Simply defining an ever-increasing repository of detects, each of which creates a stream of alerts, with an ever-increasing maintenance workload, does not scale to the point necessary to detect the most consequential campaigns. In subsequent blog posts, we will explore in more detail how the CrowdStrike Falcon®® platform’s CrowdScore technology makes dynamic decisions about whether detect rules will result in alerts, allowing much better prioritization of investigation leads, earlier detection of malicious activity, and contextualized, holistic presentation of the data.
Additional Resources
- Visit the product website to learn how the powerful CrowdStrike Falcon® platform provides comprehensive protection across your organization, workers and data, wherever they are located.
- Get a full-featured free trial of CrowdStrike Falcon® Prevent™ and see how true next-gen AV performs against today’s most sophisticated threats.